Special Session Proposals
The use of dynamic stochastic models in different areas has been increasing in recent years. In particular, Continuous Time models arise naturally for describing physical/economics/social phenomena that are observed on irregularly spaced time grids. Theoretical advantages have not been yet fully exploited in practice. Indeed, in recent literature we still find applications mainly based on discrete-time models partially due to the well- established estimation procedures. This special session is devoted to the collection of the latest developments related to the class of Continuous-Time models. In particular, papers focused on but not limited to the following topics of interest are welcome:
- Time-series forecasting model for Coronavirus outbreak and other epidemics
- Estimation and Simulation Algorithms for Continuous Time Models.
- New Continuous Time Models and related statistical properties of estimators.
Organizers:
Lorenzo Mercuri is an Associate Professor at the University of Milano. He holds a PhD in Mathematical Finance from the University of Milano-Bicocca. He is currently involved in an international research project financed by CREST Japan. His research interests include Stochastic processes, Option Pricing and Numerical Methods applied to Finance.
Department of Economics, Management and Quantitative Methods, University of Milan, Via del Conservatorio 7 Milan, Italy.
Prof. Edit Rroji is an Assistant Professor at the University of Milano-Bicocca. She holds a PhD in Mathematical Finance from the University of Milano-Bicocca. Previously, she has worked at the University of Trieste and at Politecnico di Milano. Her research interests include the modeling of financial time series and actuarial mathematics.
Department of Statistics and Quantitative Methods, University of Milano-Bicocca, Piazza dell’Ateneo Nuovo 1 Milan, Italy.
Recently, we are dealing with a large increase of interest in the time series analysis, i.e. data evolving in time. Such data come from various sources, such as e.g., automated systems, small scale computing devices, sensor networks, and are marked by different types and degrees of uncertainty: they might be imprecise, ambiguous, may include missing values, their quality may depend on the source or conditions, etc. Soft modeling and soft computing methods enable to address uncertainty because they are less rigid than traditional approaches. The desired methodology should combine different types and aspects of uncertainty, including randomness, imprecision, ambiguity, etc. By integrating fuzzy logic, probability theory and other approaches, more robust and interpretable models and tools can be developed that better reflect the uncertainty related to the observed data. The aim of this Special Session is to bring together theorists and practitioners who apply soft methods in time series analysis and forecasting to exchange ideas and discuss new trends that enrich traditional approaches and tools. Topics of interest include but are not limited to:
Soft methods for time series; fuzzy statistics for time series; recursive processing of large data; uncertainty modeling; evolving neural and neuro-fuzzy networks; analysis of censored or missing data; nalysis of fuzzy data; zzy random variables; fuzzy regression methods; granular computing; imprecise probabilities; interval data; machine learning; possibility theory; random sets; rough sets; fuzzy-rough sets; soft computing; statistical software for imprecise data
Organizers:
Prof. Katarzyna Kaczmarek-Majer Systems Research Institute, Polish Academy of Sciences, Warsaw, Poland. Katarzyna Kaczmarek-Majer is an Assistant Professor at the Systems Research Institute of the Polish Academy of Sciences. Her research interests cover time series analysis, soft computing, computational statistics, sensor data analysis and medical applications. Some of her works have been awarded at scientific conferences. She combines effectively her theoretical research with involvement in scientific projects for medicine, environmental protection, etc. She is an Associate Editor of Journal of Intelligent and Fuzzy Systems, and a vice-coordinator of the eHealth section of Polish Information Processing Society.
Prof. Przemyslaw Grzegorzewski Faculty of Mathematics and Information Science, Warsaw University of Technology, Poland and Systems Research Institute, Polish Academy of Sciences. Przemyslaw Grzegorzewski is a Full Professor at the Faculty of Mathematics and Information Science of Warsaw University of Technology and Systems Research Institute of the Polish Academy of Sciences. His areas of expertise include mathematical statistics, statistical decisions with imprecise data, fuzzy sets, data mining, soft computing, etc. Having authored several books, he edited several volumes and special issues of scientific journals. Overall, he published more than 170 papers in scientific journals, edited volumes and conference proceedings. He is a co-founder and a member of the Executive Board of the International Conference on Soft Methods in Probability and Statistics (SMPS).
Prof. Daniel Peralta IDLab, Department of Information Technology, Ghent University - imec, Ghent, Belgium.
Daniel Peralta is a Postdoctoral Researcher at the Department of Information Technology of Ghent University. His research interests involve machine learning, time series analysis, biological imaging data analysis, biometrics, large-scale datasets and parallel and distributed computing. He has published more than 20 articles in international journals.
Motivation
Time series forecasting is an important problem in many domains such as business, industry, economics, engineering, and science. It is relevant and challenging as the time series expressing real-world phenomena are in many cases very complex and stochastic, including trends, multiple seasonality, and significant random fluctuations. Therefore, time series forecasting is an active research area that has received a considerable amount of attention from researchers and practitioners for many years.
Over the past few decades, neural networks have been successfully used in this field due to their ability to capture different patterns and high expressive power to solve non-linear stochastic forecasting problems. However, in practice, it is quite challenging to properly determine an appropriate architecture and parameters of neural networks as well as the training process and time series representation so that the resulting forecasting model can achieve sound performance for both learning and generalization. Practical applications of neural forecasting models bring additional challenges, such as dealing with big, missing, distorted, and uncertain data. In addition, interpretability, explainability, and causality are paramount qualities that neural methods should aim to achieve if they are to be applied in practice.
Scope
This Special Session focuses on neural models and their application in a diverse range of forecasting areas and problems including probabilistic and multi-step forecasting. The papers are expected to report substantive results on a wide range of neural forecasting models (variants of MLP, CNN, RNN and others), discussing their architectures and training procedures, the conceptualization of a problem, time series representation, feature engineering, critical comparisons with existing techniques, and interpretation of results. Specific attention will be given to recently developed neural forecasting models including deep and hybrid solutions.
Organizers:
Prof. Grzegorz Dudek Department of Electrical Engineering,
Czestochowa University of Technology,
42-200 Czestochowa, Al. Armii Krajowej 17, Poland,
Grzegorz Dudek received his PhD in electrical engineering from Czestochowa University of Technology, Poland, in 2003 and habilitation in computer science from Lodz University of Technology, Poland, in 2013. Currently, he is an associate professor at the Department of Electrical Engineering, Czestochowa University of Technology. He is the author of two books concerning machine learning methods for load forecasting and evolutionary algorithms for unit commitment and over 100 scientific papers. He came third in the Global Energy Forecasting Competition 2014 (price forecasting track). His research interests include pattern recognition, machine learning, artificial intelligence, and their application to practical classification, regression, forecasting and optimization
problems.
Mr. Slawek Smyl Meta
1 Hacker Way, Menlo Park, CA 94025, USA
Slawek Smyl received the M.Sc. degree in Physics from Jagiellonian University, Kraków Poland in 1988, the M.Eng. degree in Information Technology from RMIT University, Melbourne VIC Australia in 1997. He is currently a Quantitative Engineer with Meta Technologies working in the area of time series forecasting. Mr. Smyl has ranked highly in forecasting competition: he won the Computational Intelligence in Forecasting International Time Series Competition 2016, got a third place in the Global Energy Forecasting Competition in 2017, and won the M4 Forecasting Competition in 2018.
Summary
One of the crucial points in the transition to a sustainable society is the ability to manage the risks of extreme events and disasters, in particular for the advancement of adaptation to climate change. The scientific literature studies in deep the relationship between climate change and extreme weather events and the implications of these events for society and sustainable development.
Actually, the nature and severity of the impacts of climatic extremes depend not only on the extremes themselves, but also on exposure and vulnerability. The interaction of climatic, environmental and human factors can lead to impacts and disasters, so it is primary to assess the important role that non-climatic factors play in determining the impacts.
Suggested topics of this special session include but are not limited to: extreme value theory, copula functions, parametric versus nonparametric approach in extreme events analysis, deep-learning methods, time-series modelling based on LSTM.
Organizer:
Prof. Giovanni De Luca Professor of Economic Statistics, University of Naples Parthenope, www.researchgate.net/profile/Giovanni_De_Luca4
Summary
The recent circumstances, oil price fluctuations, Covid-19, etc., have caused volatile behavior in demand for energy. Therefore, the need to model and forecast energy demand and equip policymakers with information to ground their decisions on necessitates the availability and information on comparative applicability of relevant techniques. Hence, research focused on the new modeling and forecasting techniques or comparing the features of the existing ones is worth to elaborate.
Organizer:
Prof. Jeyhun I. Mikayilov Energy and Macroeconomics Department,
King Abdullah Petroleum Studies & Research Center, Office#: RC 1044, http://www.kapsarc.org .
Climate:
In climate studies causality is often invoked by claiming that a phenomenon that generate movements in one time series will later generate movements in another time series, and this latter series causes weather or climate changes. (Example: the pair, the Pacific decadal variability and El Niño).
Economics:
In economics a leading relation in one series is often used to predict changes in another (but not by causation). (Example: a decline in working hours in the industry is used to predict a coming recession in the economy.)
Tools -of- the trade:
Cross correlation analysis and all the problems associated with it. Granger causality. Sugihara causality. And my own “high- resolution lead-lag method. A comparison among the methods on a common example would be nice.
Organizer:
Prof. Knut Lehre Seip Department of Technology, Arts and Design, Oslo Metropolitan University, Oslo , Norway
Motivation and objectives for the session.
The term hydrological risk may comprise different understandings depending on the type of project/research and the risk assessment goals. Extreme high-and low-flow events, also referred to as floods and droughts, respectively, have large natural, societal, and economic impacts. On the global scale, disastrous and economic losses related to high-flow events have increased dramatically over the past decades largely due to an increase in flood-prone regions settlements. The impacts of low-flow events can be recognised in among others the sanitary sewer system, crop production and the hydropower sectors. To mitigate the societal impact of hydrological and hydraulic extremes, knowledge of the processes leading to these extreme events is vital. Hydrological modelling is one of the main tools in this quest for knowledge but comes with uncertainties. For that it is necessary to deeply study the impact of hydrological and hydraulic models’ structure on the magnitude and timing of simulated extreme rainfall-runoff events.
This Special Issue is mainly aimed to welcome contributions to enhance the characterization and predictive capacity of extreme rainfall-runoff events. This is also aimed to achieve a sustainable maintenance and operation of urban rivers and their associated sanitary-rainwater sewer system, under high hydrological risk. For that, innovative methodologies and tools that can synchronize Historical with Real Time data is designed are welcomed.
Organizer:
Prof. José-Luis Molina IGA Research Group. Salamanca Univ, High Polytech Sch Engn, Avila Area Hydraulic Engn, Av Hornos Caleros 50, Avila 05003, Spain
Regarding academic background, Dr. José-Luis Molina has a degree in Civil Engineering, obtained in 2015, a degree in Environmental Sciences obtained in 2002 from the University of Granada (Spain) and three Masters related to the Environment and Water Management and Hydraulics. In addition, Dr. Molina obtained a PhD in Water Management in 2009 at the Geological and Mining Institute of Spain (IGME) and the University of Granada with the title "Integrated Analysis and Management Strategies of Aquifers in Semi-arid Areas. Application to the case study of the Altiplano (Murcia, SE Spain)". In the research dimension, he has worked internationally with many research groups, such as the Oxford University Centre for the Environment (OUCE), the Department of Land Water and Air at the University of California-Davis, etc. In 2010 he obtained a postdoctoral research position at the Centre for Integrated Assessment and Management of Watersheds (ICAM) at the Australian National University (ANU), and after that, another postdoctoral position at the Research Institute of Water and Environmental Engineering (IIAMA) at the Polytechnic University of Valencia (UPV). In addition, Professor Molina has about 45 publications in high impact SCI research journals such as "Journal of Hydrology", "Environmental Modelling and Software", "Water Resources Management". He holds an Associate Editor position of Journal of Hydrology (ELSEVIER Q1) as well as Editor in Chief of 2 Special Issues for the Journal Sustainability (MDPI Q2). He is also a reviewer of numerous research journals in the first quartile in the areas of Civil Engineering, Environment and Water Resources. He is also an evaluator of ANEP projects. As for teaching, he currently holds a position as Associate Professor in the Area of Hydraulic Engineering of the Department of Cartographic and Land Engineering of the University of Salamanca. This teaching has been developed mainly in the areas of Hydraulic Engineering and External Geodynamics, having at present a full-time teaching experience of about 12 years. He is currently the Director of the Research Group in Engineering and Water Management (IGA) by the University of Salamanca. He is currently the Director of the MEng “Modelización de Sistemas Hídricos” at University of Salamanca. Regarding transferring of knowledge he has been the IP of about 10 contracts since 2016 with a total amount of about 300.000 €.
Considering functional data anasysis (FDA) being one of the important research fields in Statistics, whether there is a spcial session with tile " Functional time series analysis and application"
Organizer:
Prof. Nengxiang Ling Hefei University of Technology, Hefei, China
He is the Guest Editor of following two Special Issues: 1. Advances in Time Series Analysis (Sensors, MDPI, Impact Factor: 3.58, Q1): https://www.mdpi.com/journal/sensors/special_issues/A_TSA 2. Artificial Intelligence and Sustainability (Sustainability, Impact Factor: 3.25, Q1): https://www.mdpi.com/journal/sustainability/special_issues/sustai_artificalintelligence
For the Special Issue in Advances in Time Series Analysis (Sensors, MDPI), the message from the Guest Editors is:
"Time series analysis has recently attracted wide attention
in many fields of science, such as remote sensing,
hydrology, geodesy, geophysics, astronomy, finance, and
medicine. Time series analysis is a very challenging task
and often requires pre-knowledge of the data. For example,
time series obtained from Earth observation data are oen
unevenly sampled (equally spaced) and have uncertainties
due to various reasons, such as sensor defects and
atmospheric effects. Therefore, new techniques that can
consider such uncertainties, as well as irregularities in
sampling, are highly demanded.
In this Special Issue, we welcome:
1) Manuscripts describing applications of the methods
mentioned above for analyzing time series obtained from
various sensors;
2) Manuscripts demonstrating new time series analysis
techniques and/or applications of existing methods."
More information in this flyer
Organizer:
Prof. Ebrahim Ghaderpour University of Calgary, 2500 University Dr. NW
Calgary, Alberta, Canada
T2N 1N4
He is the Guest Editor of following Special Issue: 1. Call for Special Issue "Inclusive Science of the Total Environment" (Journal: Sustainability) https://www.mdpi.com/journal/sustainability/special_issues/Total_Environment
For the Special Issue in Inclusive Science of the Total Environment (Sustainability MDPI), the message from the Guest Editors is:
"In the wake of persistent global push against climate change and other environmental related challenges confronting human existence in the 21st century, diverse policy drive are being recommended toward attaining a sutainable environment.
Considering the complexity of the subject of environmental sustainability, this discourse remains an evolving subject because of the inter-connectednes of the environmental aspects (including ecological systems) with all human aspects (including science and socioeconomic activities). Thus, it suffice to suggest that ‘Science of the Total Environment’ is a necessary pathway to understanding environmental sustainability. More importantly, the ‘inclusivity of Science of the Total Environment’ becomes a necessary and sufficient pathway to unearth age-long environmental challenges.
Indicatively, the attainability of a healthy state of the environment is contingent on not only natural activites, but extensively on humans’ environmental responsibility, scientific and technological advancement, and socioecomically just factors (Intergovernmental Panel on Climate Change (IPCC, 2020); United Nations Environmental Programme (UNEP)). In specific, the entire state of the environment has been largely associated with the inclusivity of the 17 Sustainable Development Goals (SDGs) of the United Nations Development Programme (UNDP) (UNDP, 2020). This account for the reason the aspects of the state of the environment has consistently been associated with health, waste management and pollutant emission across the sectors (Bekun, Alola & Sarkodie, 2019), human behaviour and organizational practice (Cop, Alola & Alola, 2020), societal and cultural norms (Aldieri et al., 2019), agricultural practices (Alola & Alola, 2018), and many more.
In essence, this special issue is geared toward inviting scientific contributions from authors on the subject that addresses the importance of inclusivity in attaining a desirable state of the total environment. An expected manuscriopt should address but tot limited to the sub-areas of health, waste management, land, pollutant emission, carbon capture and sequensation, energy, human behaviour, and innovation.."
Organizer:
Dr. Andrew Adewale Alola Department of Economics and Finance, Istanbul Gelisim University, Istanbul 34310, Turkey
Interests: economics; energy, environmental economics; fuzzy set and logic
The cryptocurrency market, or rather more generally the cryptoasset market, is a field that is rapidly and steadily growing. Similarly, the literature regarding this topic has also enormously grown over the last few years. What distinguishes this market from the tradidional financial market is that it operates 24/7 and every day, contrarily to the majority of traditional financial markets that operate within particular hours and only on business days. Moreover, what we already know about cryptoasset market is that it is characterized by wide heterogeneity, in terms of different aspects - both from the perspective of the supply and the demand.
Most of the studies and most of the available methodologies for the analysis of the behavior of cryptoasset prices is dedicated mainly for the data of daily frequency. Therefore, the literature using the daily cryptoasset data is already quite exhausted. On the other hand, there are not many studies that make use of high-frequency data. It is important to distinguish the aggregated high-frequency data (e.g. the data that contain weighted-average of prices/volumes from multiple major cryptocurrency exchanges, available for instance on coinpaprika.com up to 5-min frequency) and the tick-to-tick on-exchange data that are also openly available. Therefore, such availability of high-frequency tick-to-tick data gives a great opportunity to not only develop the cryptoasset research field but also the field of high-frequency financial data in general, which is constantly growing over the last decade or two.
Therefore, the aim of this session is to discuss the studies that focus on the usage of high-frequency cryptoasset data, since this field requires more attention in the literature because of the large gaps up to this point.
Organizer:
Mr. Damian Zięba University of Warsaw, Faculty of Economic Sciences, Department of Quantitative Finance, Warsaw, Poland.
Special Session Proposals. Previous Edition (2021)
Epidemic is a rapid and wide spread of infectious disease threatening many lives and economy damages. It is important to fore-tell the epidemic lifetime so to decide on timely and remedic actions. These measures include closing borders, schools, suspending community services and commuters. Resuming such curfews depends on the momentum of the outbreak and its rate of decay. Being able to accurately forecast the fate of an epidemic is an extremely important but difficult task. Due to limited knowledge of the novel disease, the high uncertainty involved and the complex societal-political factors that influence the widespread of the new virus, any forecast is anything but reliable. Another factor is the insufficient amount of available data. Data samples are often scarce when an epidemic just started. With only few training samples on hand, finding a forecasting model which offers forecast at the best efforts is a big challenge in machine learning. This section invites works and works-in-progress from both academia and industrial partners to share and present ideas which could contribute to understanding and hopefully subsiding this outbreak which has evolved to global pandemic.
Papers related but not limited to the following topics of interest are solicited:
- Time-series forecasting model for Coronavirus outbreak and other epidemics
- Machine learning, AI and Big Data for modeling epidemic outbreaks
- Decision support tools and optimization for modelling and controlling epidemics
- Hybrid models of forecasting and other states-of-arts for predicting epidemics
- Social media and text mining for predicting lifetimes and trends of epidemics
- Data analytics and correlations of past epidemics and Coronavirus outbreak
- Forecasting of post-epidemic stock markets and worldwide economy impact
- Other issues related to the epidemics: Government policy, medical resources, etc.
Organizers:
Prof. Simon James Fong, University of Macau, Macau SAR.
Prof. Nilanjan Dey, Techno India College of Technology, India
Prof. Rubén González Crespo, Universidad Internacional de La Rioja, Logroño, Spain
Prof. Enrique Herrera-Viedma, University of Granada, Spain
Prof. Antonio J. Tallón-Ballesteros, University of Huelva, Huelva, Spain
MOTIVATION
Over the past few decades, application of simple statistical procedures with considerable heuristic or judgmental input was the beginning of forecasting, then in the 80’s, sophisticated time series models started to be used by some of the dynamic system operators, and these approaches, were to become pioneering works in this field. Soft computing methods including support vectors regression (SVR), fuzzy inference system (FIS) and artificial neural networks (ANN) to time-series forecasting (TSF) has been growing rapidly in order to unify the field of forecasting and to bridge the gap between theory and practice, making forecasting useful and relevant for decision-making in many fields of the sciences. The purpose of this session is to hold smaller, informal meetings where experts in a particular field of forecasting can discuss forecasting problems, research, and solutions in the field of automatic control. There is generally a nominal registration fee associated with attendance. This session aims to debate in finding solutions for problems facing the field of forecasting. We wish to hear from people working in different research areas, practitioners, professionals and academicians involved in this problematic.
SCOPE
The session seeks to foster the presentation and discussion of innovative techniques, implementations and applications of different problems that are Forecasting involved, specially in real-world problems applied to control and automation.
• Time Series Analysis
• Time Series Forecasting
• Evaluation of Forecasting Methods and Approaches
• Forecasting Applications in Business, Energy and Price Demand, Hydrology, etc.
• Impact of Uncertainty on Decision Making
• Seasonal Adjustment
• Multivariate Time Series Modelling and Forecasting
• Marketing Forecasting
• Economic and Econometric Forecasting
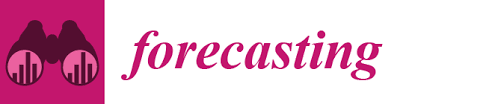
Dr.Cristian Rodriguez, the Guest Editor of the Special Issue on "Bayesian Time Series Forecasting" at the Journal of Forecasting and the organizer of the Special Session in ITISE-2022: "SS2. Computational Intelligence for Applied Time Series Forecasting in Complex Systems (CIATSFCS)".
Organizers:
Prof. Cristian Rodriguez Rivero,University of Amsterdam, c.m.rodriguezrivero@uva.nl, IEEE CIS, Co-Founder LA-CIS.
Prof. Alvaro Orjuela Cañón,Universidad del Rods, dorjuelaco@ieee.org, IEEE CIS, Co-Founder Board of Directors of LA-CIS.
Prof. Héctor Daniel Patiño,Universidad Nacional de San Juan, Argentine,dpatino@inaut.unsj.edu.ar. IEEE CIS.
Prof. Julián Antonio Pucheta,Universidad Nacional de Córdoba, Argentine,jpucheta@efn.uncor.edu.
Prof. Gustavo Juarez,Universidad Nacional de Tucumán, Argentine, juarez.gustavo@ieee.org, IEEE CIS.
Prof. Leonardo Franco,School of Engineering in Informatics, University of Malaga, Spain. lfranco@lcc.uma.es. IEEE CIS.
Organizer:
Prof. Pitshou Bokoro,Head of Department: Electrical and Electronic Engineering Technology, University of Johannesburg
Control charts have gradually been approved in pioneer industries as effective tools used in statistical process control (SPC) to ensure quality and save manufacturing costs. It is mainly used to identify the change in the process before manufacturing nonconforming items in massive amounts. After introducing the basic theory of process monitoring by Shewhart, numerous control charts have been developed to achieve special objectives under various assumptions [1]. One of the main assumptions of statistical process monitoring (SPM) is that the sampled observations at different time points must be independent. Nevertheless, the independence assumption is not realistic from two types of practical experiences: (1) sampling in high frequency induce autocorrelation in some processes, and (2) sampling from processes, such as chemical and environmental that introduce inherent autocorrelation [2]. In fact, in some industrial/non-industrial processes (e.g., continuous manufacturing processes, financial processes, health care systems, environmental phenomena, network monitoring), a correlation exists among adjacent observations [3]. The autocorrelation, if ignored, can significantly influence the statistical features of traditional control charts. This has led to the extension of various charts for autocorrelated observations. Two model-based approaches can be used to treat the process when the serial correlation exists among observations. These approaches include the residual control charts and the modified control charts [4]. In the first approach, the control charts are applied to the residuals obtained after fitting a time series model to eliminate the correlated structure. The special cause chart (SCC) was developed by Alwan and Roberts [5] as an initial study in this approach. In the second approach, the correlated observations are directly used on the control charts in which the control limits are adjusted according to the autocorrelation structure. Vasilopoulos and Stamboulis [6] performed the initial study on proposing the modified control chart. In addition to the introduced approaches, neural network-based control charts can also be categorized as the third approach, in which the data are processed without the requirements of identifying models or making adjustments [7]. In the fourth approach, some sampling strategies are taken to reduce the effect of autocorrelation [8]. Among the recent studies using different approaches, the reader can refer to [9-12]. All studies on developing control charts for autocorrelated data seem to have their advantages and disadvantages. Therefore, it is necessary to present a more simple and effective SPC methodology for monitoring autocorrelated processes. Moreover, the existing approaches can be applied in practice. Environmental & social sciences, finance, renewable sources, manufacturing, medicine, agriculture, etc are among the attractive fields that experience violation of independence assumption in some cases. Such applications can be treated with the introduced approaches.
Organizer:Prof. Alireza Faraz and Prof. Samrad Jafarian-Namin,Industrial Engineering Department Faculty of Engineering Yazd University, Yazd, Iran
Forecasting high-dimensional data and analysis of time series with hundreds/thousand of attributesinations) is challenging problem, with application in multiple problems of real life (problems in economy, energy, climate, etc). Real problems in which you have to predict / analyze or treat large volumes of data are welcome to this session.
Organizer:
Prof. Luis Javier Herrera ,Dep. Computer Architecture and Computer Technology, University of Granada, Spain
Prof. Fernando Rojas ,Dep. Computer Architecture and Computer Technology, University of Granada, Spain
Within the field of science and engineering, it is very common to have data arranged in the form of time series data which must be subsequently analyzed, modeled and classified with the eventual goal of predicting future values. The literature shows that all these tasks related to time series can be undertaken using computational intelligence methods. In fact, new and further computational intelligence approaches, their efficiency and their comparison to statistical methods and other fact-checked computational intelligence methods, is a significant topic in academic and professional projects and works. Therefore, this special session aims at showing to our research community high quality and state of the art computational intelligence (and statistical) related works, applied to time series data and their tasks: analysis, forecasting, classification, and clustering. Furthermore, the experts can, from the starting point that the works shown provide, discuss different solutions and research issues for these topics.
Organizer:
Prof. Héctor Pomares ,Dep. Computer Architecture and Computer Technology, University of Granada, Spain
Prof. German Gutierrez,Dep. Computer Science, E.P.S. University Carlos III of Madrid, Spain
The present outbreak of COVID-19 disease, caused by the SARS-CoV-2 virus, has put the planet in quarantine. On January 30, 2020, the World Health Organization (WHO) declared the COVID-19 outbreak a "public health emergency of international concern”, and a pandemic on March 11. Due to the rapid spread of the disease and the changing nature of diagnosis protocols, the official counts worldwide severely miss-report the true number of infected individuals. This is a common feature of epidemiological data. On the other hand the extreme measures taken to curb the rate of infection have negatively impacted the economy. This session invites works reporting solutions to these two important problems related to COVID-19: what was not seen and how it has hurt our finances.
Organizer:
Prof. Argimiro Arratia ,Universitat Politécnica de Catalunya Dept. of Computer Science, Barcelona
Alejandra Cabaña , Universitat Autònoma de Barcelona, Dept. de Matemàtiques.
Providing your health care was a new area of supply and a valuable tool for preventing future health events or situations, such as: B. the leadership of the health service and the need for health care. It facilitates preventive medicine and intervention strategy in the health sector and informs in advance of the health operation so that it is possible to adopt adequate mitigation measures to the minimum risk and command management.
Organizer:
Prof. J. Wang ,The Monash University, Australia
This session aims at presenting the recent developments of Time Series Modelling applied to financial and energy futures data. In particular, a focus is on studies that develop and apply recent nonlinear econometric models to reproduce financial market dynamics, capture financial data properties (asymmetry, volatility clustering, kurtosis excess, nonmorality, etc.). Papers on high frequency data and nonparametric econometric models are particularly welcomed.
Organizer:
Prof. H. Zhag ,University of York, Uk
It is well-known that time series analysis is time-consuming when large data sets are used, soft computing methods being recommended for obtaining a balance between the models' accuracy and speed of solving the problem at hand. Therefore, this special session aims to present the advances in the fields of modeling the hydro-meteorological time series and water quality assessment. Submissions reflecting theoretical methods and experimental works in the field of statistical analysis and applications to modeling hydro-meteorological time series, water quality assessment are expected.
Suggested topics of this special session include but are not limited to: o Parametrical versus non-parametric approaches in hydro-meteorological data modeling o Critical evaluation and comparisons of alternative approaches for hydro-meteorological series modeling o New techniques for spatial data analysis applied to hydro-meteorology o New software for data analysis – development and applications to hydro-meteorological time series o Soft computing and fuzzy techniques in water hydro-meteorological time series modeling o Forecasting hydro-meteorological series o New techniques for water quality evaluation, monitoring, and forecast
Organizer:
Prof. dr. habil. Alina Bărbulescu , Technical University of Civil Engineering, Bucharest
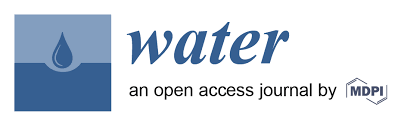
Prof. dr. habil. Alina Barbulescu, the Guest Editor of the Special Issue on "Assessing Hydrological Drought in a Climate Change: Methods and Measures" at the Journal WATER and the organizer of the Special Session in ITISE-2022: "SS11. Advances in hydro-meteorological time series analysis and forecasts.".
Organizer:
Prof. Dr. Dwivedi, R, , M N Natl Inst Technol Allahabad, Geog Informat Syst Cell, Allahabad 211004, Uttar Pradesh, India.
Causal Reasoning (CR) should be seen as a reasoning pattern whose main goal is to predict the consequences or effects of some previous factors (Pearl, J., 2009). For instance, a joint distribution PB specifies the probability PB (A = a |E = e) of any event a given any observations e. The probability of the event a is computed by summing the probabilities of all of the entries in the resulting posterior distribution that are consistent with a. Queries such as these, where it is about the prediction of “downstream” effects of various factors, are instances of causal reasoning or prediction.
Causality in hydrological records has not been deeply studied and it could be done by means of the joint use of different forms of reasoning patterns. These forms are Causal Reasoning (CR), Evidential Reasoning (ER) and Intercausal Reasoning (IR) (Koller and Friedman, 2009; Pearl, J., 2009). CR is used when the approach is done from top to bottom. In this sense, the analysis is focused on the cause and the objective comprises the prediction of the effect or consequence. Consequently, the queries in form of conditional probability, where the “downstream” effects of various factors are predicted, are instances of causal reasoning or prediction. ER comprises bottom-up reasoning, so the analysis is focused on the consequence (effect) and the cause is inferred (Bayesian Inference). IR is probably the hardest concept to understand. It comprises the interaction of different causes for the same effect. This type of reasoning is very useful in Hydrology, where a consequence can be generated or explained from several causes. Furthermore, one of the most exciting prospects in recent years has been the possibility of using the theory of Bayesian Networks to discover causal structures in raw data (Historical runoff record) (Pearl, J., 2014). This is performed through the usage of historical runoff data to train and populate the BN implementation. Consequently, AI techniques such as CR and ER and/or IR provide new horizons for this type of studies
Furthermore, temporal dependence of hydrological time series has been deeply studied through classic and new approaches (Hao and Singh, 2016; Mishra and Singh, 2010; Mishra and Singh, 2011; Molina et al., 2016; Molina and Zazo, 2017). Conversely, spatial and spatio-temporal dependence for hydrologic science and engineering is much poorer studied (Holmström et al., 2015; Macián-Sorribes et al., 2020) and even more through Bayesian approaches (Lasinio et al., 2007; Wikle et al., 1998). This is because of some reasons explained as follows: complexity of characterizing and differentiating water sub-systems, scarcity of spatial data availability, difficulties in the application of spatial statistical methods, among others. Consequently, there is a general clear necessity of strengthening the spatio-temporal dependence studies on water systems (Holmström et al., 2015) and multipurposes through Causal Reasoning Modelling.
Organizers:
Dr. José-Luis Molina , IGA Research Group (University of Salamanca).
Dr. Santiago Zazo
Dr. Ana María Martín Casado
Dra. Carmen Patino
Dr. Fernando Espejo
D. Abedin Hosseinpour
Recent years have seen a rapid increase in the availability of high-dimensional time series in diverse contexts such as web traffic, sensor networks, finance, econometrics, neuroimaging, functional genomics, and more. To facilitate theory and computations, statistical methods for high-dimensional time series crucially rely on the concepts of sparsity, parsimony, and dimension reduction. Regularization methods based on LASSO, SCAD, and MCP penalties, for example, are widely used to induce sparsity in high-dimensional regression and covariance estimation. Dynamic factor models constitute a popular approach to reducing the dimension of time series. Nonstationarity, a problem that often compounds the high dimensionality of time series, can be tackled with locally stationary models, regime-switching models, latent process models, and segmentation methods.
This session aims to attract novel theoretical and methodological contributions to the analysis of high-dimensional time series. In addition to the aforementioned topics, themes of interest include but are not restricted to: prediction, forecasting, variable selection, classification, change point detection, low rank + sparse methods, and spectral domain analysis.
Organizer:
Dr. David Degras , Assistant Professor, Department of Mathematics, University of Massachusetts Boston, web: um-boston.academia.edu/DavidDegras
David Degras received his PhD in Statistics from the Université Paris 6, France, in 2008. He was a Postdoctoral Researcher at the Statistical and Applied Mathematical Sciences Institute (SAMSI) in 2010-11 and served as an Assistant Professor in the Department of Mathematical Sciences at DePaul University from 2011 to 2016. He is currently an Assistant Professor in the Department of Mathematics at the University of Massachusetts Boston. His research interests include computational statistics, convex and combinatorial optimization, neuroimaging, statistical learning, and functional data analysis.